Understanding Inferential Statistics: A Psychological Perspective
In the realm of psychology, inferential statistics occupies a pivotal role, enabling researchers to delve beyond the immediate data in front of them and make broader generalizations. It empowers us to extract meaningful insights from limited samples, allowing us to draw conclusions about the wider population.
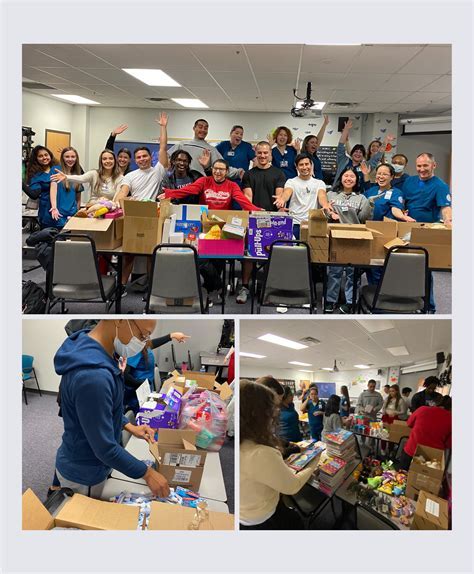
The Essence of Inferential Statistics: Extrapolating Knowledge
Inferential statistics stands as a crucial branch of statistics, focused on drawing inferences about a larger population based on a smaller sample. It involves employing statistical methods to test hypotheses and determine the probability of obtaining specific results, thus painting a clearer picture of the underlying phenomena.
Key Concepts in Inferential Statistics: Laying the Foundation
To fully grasp inferential statistics, it is essential to delve into its fundamental concepts:
-
Hypothesis Testing: Formulating a hypothesis, collecting data, and analyzing it to determine whether the hypothesis is supported or rejected.
-
Confidence Intervals: Constructing a range of values within which the true population parameter is likely to fall.
-
Statistical Significance: Determining the likelihood of obtaining the observed results due to chance or meaningful differences.
-
Sample Size and Power: Understanding the optimal sample size that yields meaningful results and the likelihood of detecting true effects.
Applications of Inferential Statistics: Unveiling Psychological Insights
Inferential statistics proves invaluable in a myriad of psychological applications, empowering researchers to:
-
Conduct Surveys: Gauging the opinions and attitudes of a larger population based on a representative sample.
-
Test Psychological Theories: Examining the validity of psychological theories by testing hypotheses derived from them.
-
Evaluate Treatment Effectiveness: Determining the efficacy of psychological interventions by comparing treatment groups to control groups.
-
Predict Individual Outcomes: Forecasting future behaviors or characteristics of individuals based on observed data.
Inferential Statistics in Action: Illustrating its Power
Let’s consider a scenario where a researcher aims to determine if a particular therapy is effective in reducing anxiety levels. The researcher randomly assigns participants to either a therapy group or a control group. After the therapy period, the anxiety levels of both groups are measured.
Using inferential statistics, the researcher calculates a t-test to compare the mean anxiety levels of the two groups. The t-test reveals a significant difference, indicating that the therapy group experienced a statistically significant reduction in anxiety compared to the control group.
Benefits of Inferential Statistics: Advancing Psychological Knowledge
Inferential statistics offers a multitude of benefits for psychological research:
-
Extrapolating Results: Enables researchers to generalize their findings beyond the sample studied.
-
Hypothesis Testing: Provides objective evidence to support or refute hypotheses, enhancing the credibility of findings.
-
Data Interpretation: Aids in making sense of complex data by identifying patterns and relationships.
-
Prediction and Intervention: Facilitates the development of predictive models and effective interventions based on research findings.
Limitations of Inferential Statistics: Addressing Concerns
Despite its power, inferential statistics has certain limitations:
-
Sample Representativeness: Inferences rely on the assumption that the sample accurately represents the larger population.
-
Sampling Error: Results can be influenced by the random sampling process, leading to some degree of uncertainty.
-
Significance Threshold: The use of a significance threshold can lead to false positives or false negatives.
-
Data Interpretation: Misinterpreting statistical results or overgeneralizing findings can occur without careful consideration.
Innovative Applications: Unveiling New Frontiers
The field of inferential statistics is constantly evolving, with innovative applications emerging. One such concept is Bayesian statistics, which allows for continuous updating of inferences as new data becomes available.
Tables: Summarizing Key Information
Concept | Definition |
---|---|
Hypothesis Testing | Formulating and testing hypotheses based on data |
Confidence Intervals | Range of values containing the true population parameter |
Statistical Significance | Probability of results occurring by chance |
Sample Size and Power | Optimal sample size for meaningful results |
Research Question | Hypothesis | Statistical Test |
---|---|---|
Is therapy effective in reducing anxiety? | Therapy group will have lower anxiety levels than control group. | t-test |
Do gender and age predict job satisfaction? | Gender and age have significant effects on job satisfaction. | Multiple regression analysis |
Does mindfulness training improve cognitive function? | Mindfulness training group will have better cognitive performance than control group. | ANOVA |
Data Type | Statistical Test |
---|---|
Continuous: Normally distributed | t-test, z-test, analysis of variance (ANOVA) |
Continuous: Not normally distributed | Mann-Whitney U test, Kruskal-Wallis test |
Categorical: Binary | chi-square test |
Categorical: Multiple | logistic regression, multinomial logistic regression |
FAQs: Answering Common Questions
-
What is the difference between descriptive and inferential statistics?
– Descriptive statistics summarize and describe data, while inferential statistics draw inferences about a larger population based on a smaller sample. -
When should I use inferential statistics?
– Use inferential statistics when you want to make generalizations beyond the sample you have or test hypotheses about a population. -
How do I choose the right statistical test for my data?
– The appropriate statistical test depends on the type of data (continuous, categorical, etc.) and the research question being investigated. -
What is statistical significance and how do I interpret it?
– Statistical significance indicates the probability of obtaining the observed results due to chance. A result is considered statistically significant when this probability is low (usually less than 0.05). -
What is a confidence interval and how do I use it?
– A confidence interval estimates the range within which the true population parameter is likely to fall. It allows us to make statements about the population with a certain level of confidence. -
What are the limitations of inferential statistics?
– Inferential statistics relies on assumptions about the sample and the population, and it can be affected by sampling error and misinterpretations. -
How can I improve my inferential statistical skills?
– Take courses, read books and articles, practice using statistical software, and seek guidance from experts. -
What are the ethical considerations in using inferential statistics?
– Use inferential statistics responsibly, avoid overgeneralizing results, and disclose any limitations or biases in your research.