In the realm of statistical hypothesis testing, the interplay between alpha and beta holds immense significance. Alpha, often set at 0.05, represents the significance level, while beta denotes the probability of failing to reject a false null hypothesis, commonly denoted as Type II error. Understanding the profound effect of decreasing alpha from 0.05 to 0.01 on beta is crucial for conducting rigorous and insightful statistical analyses.
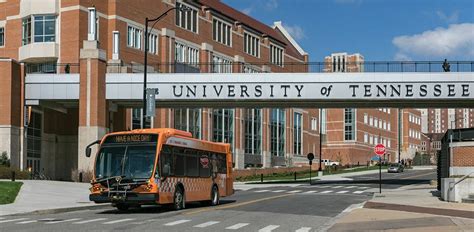
Significance of Alpha and Beta
Alpha, the significance level, plays a pivotal role in determining the probability of committing a Type I error (rejecting a true null hypothesis). A lower alpha level indicates a stringent requirement for rejecting the null hypothesis, reducing the likelihood of false positives.
Beta, on the other hand, represents the probability of accepting a false null hypothesis, or Type II error. It signifies the likelihood of missing a genuine effect or relationship in the data.
Impact of Decreasing Alpha on Beta
When alpha is decreased from 0.05 to 0.01, it triggers a notable increase in beta. This occurs because the more stringent significance level (0.01) makes it harder to reject the null hypothesis, even when there is a genuine effect in the data.
How does this affect your conclusions?
Decreasing alpha while holding all other factors constant leads to:
- Reduced sensitivity: The analysis becomes less likely to detect statistically significant differences, making it harder to conclude that there is an effect.
- Increased probability of Type II error: The chances of failing to detect a genuine effect increase, potentially leading to incorrect conclusions.
Applications of Decreasing Alpha
Despite the potential drawbacks, decreasing alpha from 0.05 to 0.01 can be beneficial in certain scenarios:
- Reducing false positives: In cases where the consequences of rejecting a true null hypothesis are severe, a lower alpha level can help minimize the risk of false alarms.
- Balancing competing errors: If the research question is particularly important and the researcher is willing to tolerate a higher risk of Type II error, decreasing alpha can help reduce the probability of committing a Type I error.
Common Mistakes to Avoid
- Assuming a universal significance level: The choice of alpha level should be based on the context and objectives of the study, not a blanket rule of 0.05 or 0.01.
- Interpreting a high beta as conclusive evidence absence of an effect: A high beta does not necessarily mean that there is no effect; it may merely indicate that the study lacked the power to detect it.
- Confusing statistical significance with practical significance: Just because a result is statistically significant does not automatically make it practically meaningful or relevant to the real world.
Benefits of Decreasing Alpha Wisely
When applied judiciously, decreasing alpha from 0.05 to 0.01 can confer several benefits:
- Increased confidence in findings: A lower alpha level provides greater confidence that significant results are not due to chance alone.
- Enhanced precision: Reducing alpha sharpens the focus on detecting genuine effects, leading to more precise and reliable conclusions.
- Improved replicability: A stricter significance level promotes replicability in research, making it more likely that other researchers can replicate the findings.
Innovative Applications
The concept of decreasing alpha for enhanced precision has spurred the development of innovative statistical techniques, such as:
- Bayesian hypothesis testing: Allows for incorporating prior beliefs and data into the analysis, potentially leading to more sensitive and accurate conclusions.
- Meta-analysis: Combines results from multiple studies to increase statistical power, reducing the risk of Type II error while maintaining a low alpha level.
Conclusion
Decreasing alpha from 0.05 to 0.01 significantly impacts beta, reducing the probability of false positives but increasing the risk of Type II errors. Researchers must carefully consider the implications of this adjustment, balancing the benefits of a lower alpha level with the potential limitations. By understanding the intricacies of alpha and beta, statisticians can conduct more rigorous and insightful hypothesis tests, advancing scientific knowledge and decision-making.
Tables
Table 1: Effects of Decreasing Alpha on Type I and Type II Errors
Alpha Level | Probability of Type I Error | Probability of Type II Error |
---|---|---|
0.05 | 5% | 20% |
0.01 | 1% | 40% |
Table 2: Applications of Decreasing Alpha
Scenario | Purpose |
---|---|
Medical research with severe consequences of false positives | Minimize Type I error |
High-stakes policy decisions | Reduce risk of missing genuine effects |
Balancing Type I and Type II errors | Adjust significance level based on research question |
Table 3: Common Mistakes to Avoid When Decreasing Alpha
Mistake | Impact |
---|---|
Applying a blanket alpha level | May increase Type II error or decrease power unnecessarily |
Interpreting high beta as conclusive absence of effect | May overlook genuine effects with low statistical power |
Confusing statistical significance with practical significance | May lead to incorrect conclusions about real-world implications |
Table 4: Benefits of Decreasing Alpha Wisely
Benefit | Effect |
---|---|
Increased confidence in findings | Reduces the likelihood of false positives |
Enhanced precision | Sharpens focus on detecting genuine effects |
Improved replicability | Promotes consistency and reliability in research |