Data analysis has emerged as a transformative force for businesses, offering unparalleled opportunities to unlock valuable insights, drive informed decision-making, and achieve tangible outcomes. With the proliferation of data in every aspect of business operations, leveraging these assets effectively has become imperative for success in today’s competitive landscape.
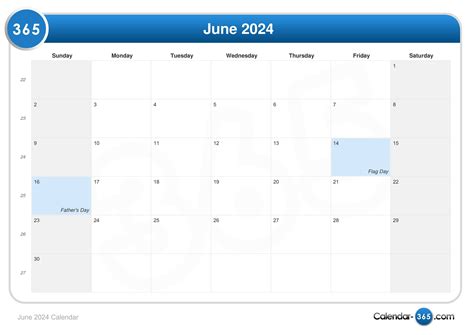
The Value of Data-Driven Insights
According to a recent study by McKinsey, data-driven organizations are 23 times more likely to acquire new customers, 6 times more likely to retain existing customers, and 19 times more likely to increase their profitability. These staggering figures underscore the immense value that data analysis can bring to businesses.
By systematically collecting, cleaning, and analyzing data, businesses can:
- Identify trends and patterns: Uncover hidden insights that provide a deeper understanding of customer behavior, market dynamics, and industry trends.
- Predict future outcomes: Utilize predictive analytics to anticipate demand, optimize inventory levels, and forecast financial performance.
- Optimize operations: Analyze operational data to identify inefficiencies, streamline processes, and reduce costs.
- Personalize customer experiences: Leverage customer data to tailor products, services, and marketing campaigns to individual needs.
- Drive innovation: Extract novel ideas and develop innovative products and services that meet emerging market demands.
Benefits of Data Analysis for Businesses
The benefits of data analysis extend far beyond the immediate insights it generates. It empowers businesses to:
- Improve decision-making: Data-driven decisions are based on objective evidence, reducing guesswork and improving the likelihood of success.
- Enhance customer satisfaction: Deeper insights into customer preferences and behavior enable businesses to provide personalized experiences that drive loyalty.
- Increase operational efficiency: Optimizing operations based on data leads to reduced costs, improved productivity, and increased profitability.
- Gain competitive advantage: Data analysis provides a competitive edge by identifying new opportunities, understanding market trends, and staying ahead of the competition.
- Foster innovation: Data analysis sparks creativity and fosters innovation by providing a foundation for developing new products, services, and business models.
Common Mistakes to Avoid in Data Analysis
Despite its immense potential, data analysis can be fraught with pitfalls if certain mistakes are not avoided. Common errors include:
- Lack of clear objectives: Failing to define clear goals before analyzing data can lead to meaningless or irrelevant insights.
- Ignoring data quality: Poor-quality data can skew results and undermine the validity of conclusions.
- Overreliance on automated tools: While automated tools can assist in data analysis, they cannot replace human expertise and critical thinking.
- Cherry-picking data: Selecting only data that supports a desired outcome can result in biased and misleading conclusions.
- Misinterpreting results: Incorrectly interpreting data findings can lead to erroneous decisions and missed opportunities.
Overcoming Challenges in Data Analysis
To effectively overcome the challenges associated with data analysis, businesses must:
- Establish a data governance framework: Develop clear guidelines and processes for data collection, storage, and usage to ensure data quality and integrity.
- Invest in data literacy training: Provide employees with the skills and knowledge necessary to understand and interpret data analysis results.
- Foster a culture of data-driven decision-making: Encourage employees to rely on data when making decisions and allocate resources accordingly.
- Partner with data analytics experts: Seek external expertise to supplement in-house capabilities and gain access to advanced analytics techniques.
- Stay abreast of emerging technologies: Embrace new technologies such as machine learning and artificial intelligence to enhance data analysis capabilities.
Innovative Applications of Data Analysis
The potential applications of data analysis are boundless, limited only by the creativity and imagination of businesses. Here are a few examples of innovative ways to leverage data:
- Customer churn prediction: Use data to identify customers at risk of leaving and implement targeted interventions to retain them.
- Dynamic pricing: Analyze demand and cost data to adjust prices in real-time, optimizing revenue and customer satisfaction.
- Personalized product recommendations: Leverage customer data to tailor product recommendations and offer highly relevant suggestions.
- Risk assessment in financial services: Analyze financial data to assess the risk of loan applicants and optimize portfolio management strategies.
- Inventory optimization in retail: Use data to predict demand and optimize inventory levels, minimizing waste and lost sales opportunities.
FAQs
1. What is the first step in data analysis?
Defining clear objectives and understanding the business problem to be solved.
2. What are the key elements of a successful data analysis project?
High-quality data, clear objectives, skilled analysts, and a culture of data-driven decision-making.
3. What are the ethical considerations in data analysis?
Ensuring data privacy, transparency, and avoiding biased or discriminatory outcomes.
4. What are the most in-demand data analysis skills?
Technical skills such as programming, data visualization, and statistical modeling; as well as soft skills such as critical thinking, problem-solving, and communication.
5. What is the future of data analysis?
The increasing availability of data, coupled with advancements in technology, will drive the continued growth and evolution of data analysis, empowering businesses with even more powerful insights and capabilities.
6. What are the emerging trends in data analysis?
- Augmented analytics: Using artificial intelligence to enhance data analysis capabilities.
- Prescriptive analytics: Going beyond descriptive and predictive analytics to provide actionable recommendations.
- Real-time analytics: Analyzing data as it is generated for immediate insights.
- Data democratization: Making data analysis accessible to a wider range of users.
7. What are the continuing challenges in data analysis?
- Data quality issues: Ensuring the accuracy and reliability of data remains a significant challenge.
- Skilled workforce shortage: Finding and retaining qualified data analysts can be difficult for many businesses.
- Ethical and privacy concerns: Balancing the value of data analysis with the need to protect individual privacy is a critical issue.
8. What are the key metrics for measuring the success of a data analysis project?
- Improved decision-making: Tracking the impact of data analysis on business decisions.
- Increased revenue: Demonstrating the financial benefits of data analysis.
- Enhanced customer satisfaction: Measuring the impact of data analysis on customer experiences.
- Cost reduction: Quantifying the cost savings achieved through data analysis.
Conclusion
Data analysis has emerged as a powerful tool for businesses to unlock value, drive innovation, and achieve tangible outcomes. By embracing data-driven insights, organizations can gain a competitive edge, optimize operations, and create a foundation for sustainable growth. Overcoming challenges and embracing innovative applications will ensure that businesses continue to harness the transformative power of data analysis in the years to come.
Table 1: Benefits of Data Analysis for Businesses
Benefit | Description |
---|---|
Improved decision-making | Data-driven decisions are based on objective evidence, reducing guesswork and improving the likelihood of success. |
Enhanced customer satisfaction | Deeper insights into customer preferences and behavior enable businesses to provide personalized experiences that drive loyalty. |
Increased operational efficiency | Optimizing operations based on data leads to reduced costs, improved productivity, and increased profitability. |
Gain competitive advantage | Data analysis provides a competitive edge by identifying new opportunities, understanding market trends, and staying ahead of the competition. |
Foster innovation | Data analysis sparks creativity and fosters innovation by providing a foundation for developing new products, services, and business models. |
Table 2: Common Mistakes to Avoid in Data Analysis
Mistake | Description |
---|---|
Lack of clear objectives | Failing to define clear goals before analyzing data can lead to meaningless or irrelevant insights. |
Ignoring data quality | Poor-quality data can skew results and undermine the validity of conclusions. |
Overreliance on automated tools | While automated tools can assist in data analysis, they cannot replace human expertise and critical thinking. |
Cherry-picking data | Selecting only data that supports a desired outcome can result in biased and misleading conclusions. |
Misinterpreting results | Incorrectly interpreting data findings can lead to erroneous decisions and missed opportunities. |
Table 3: Innovative Applications of Data Analysis
Application | Description |
---|---|
Customer churn prediction | Use data to identify customers at risk of leaving and implement targeted interventions to retain them. |
Dynamic pricing | Analyze demand and cost data to adjust prices in real-time, optimizing revenue and customer satisfaction. |
Personalized product recommendations | Leverage customer data to tailor product recommendations and offer highly relevant suggestions. |
Risk assessment in financial services | Analyze financial data to assess the risk of loan applicants and optimize portfolio management strategies. |
Inventory optimization in retail | Use data to predict demand and optimize inventory levels, minimizing waste and lost sales opportunities. |
Table 4: Emerging Trends in Data Analysis
Trend | Description |
---|---|
Augmented analytics | Using artificial intelligence to enhance data analysis capabilities. |
Prescriptive analytics | Going beyond descriptive and predictive analytics to provide actionable recommendations. |
Real-time analytics | Analyzing data as it is generated for immediate insights. |
Data democratization | Making data analysis accessible to a wider range of users. |